pKa accurately predicts acid dissociation constants directly from structure, enabling researchers to understand ionization behavior of organic molecules.
Vendor
ACD Labs
Company Website
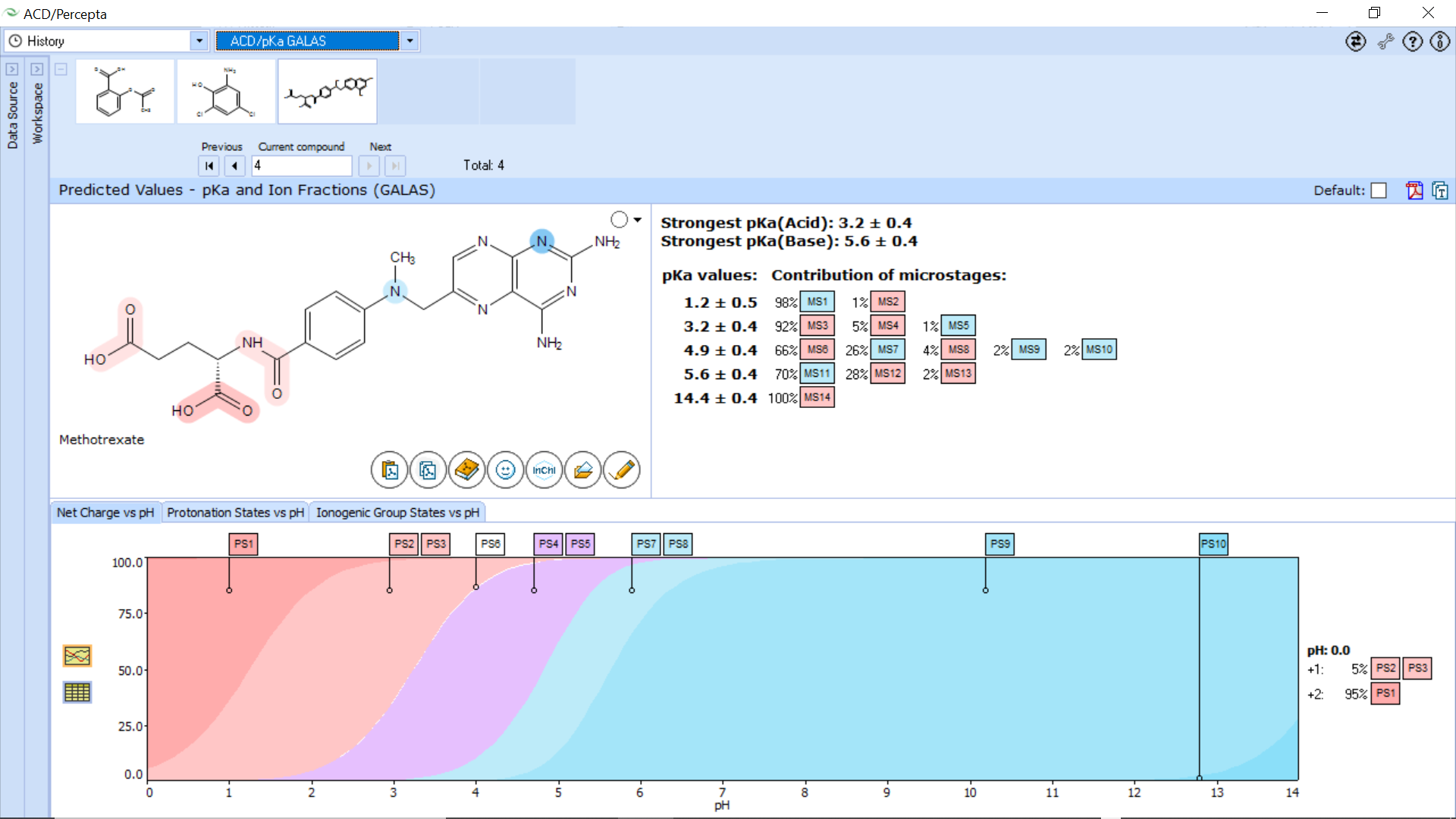
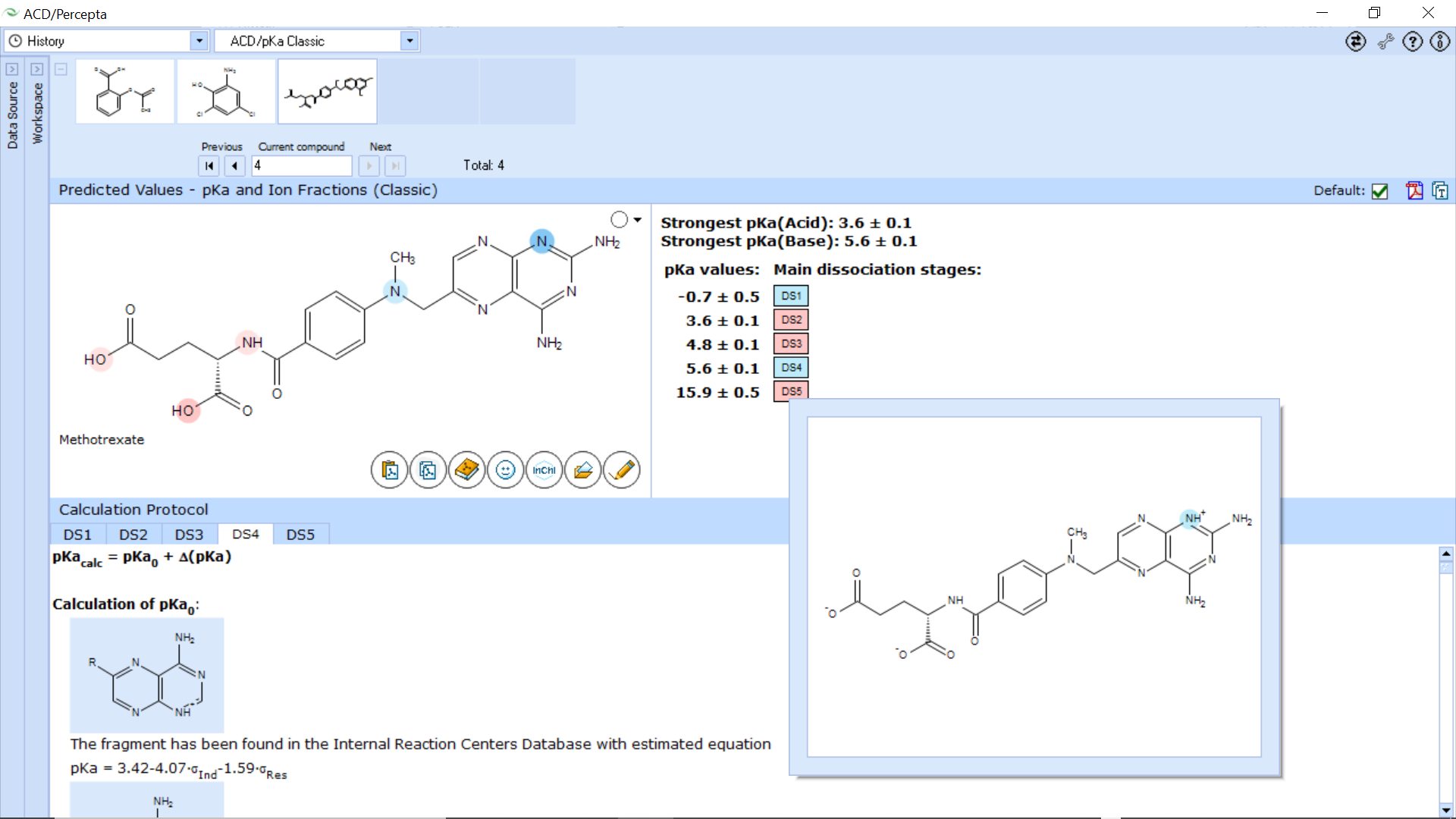
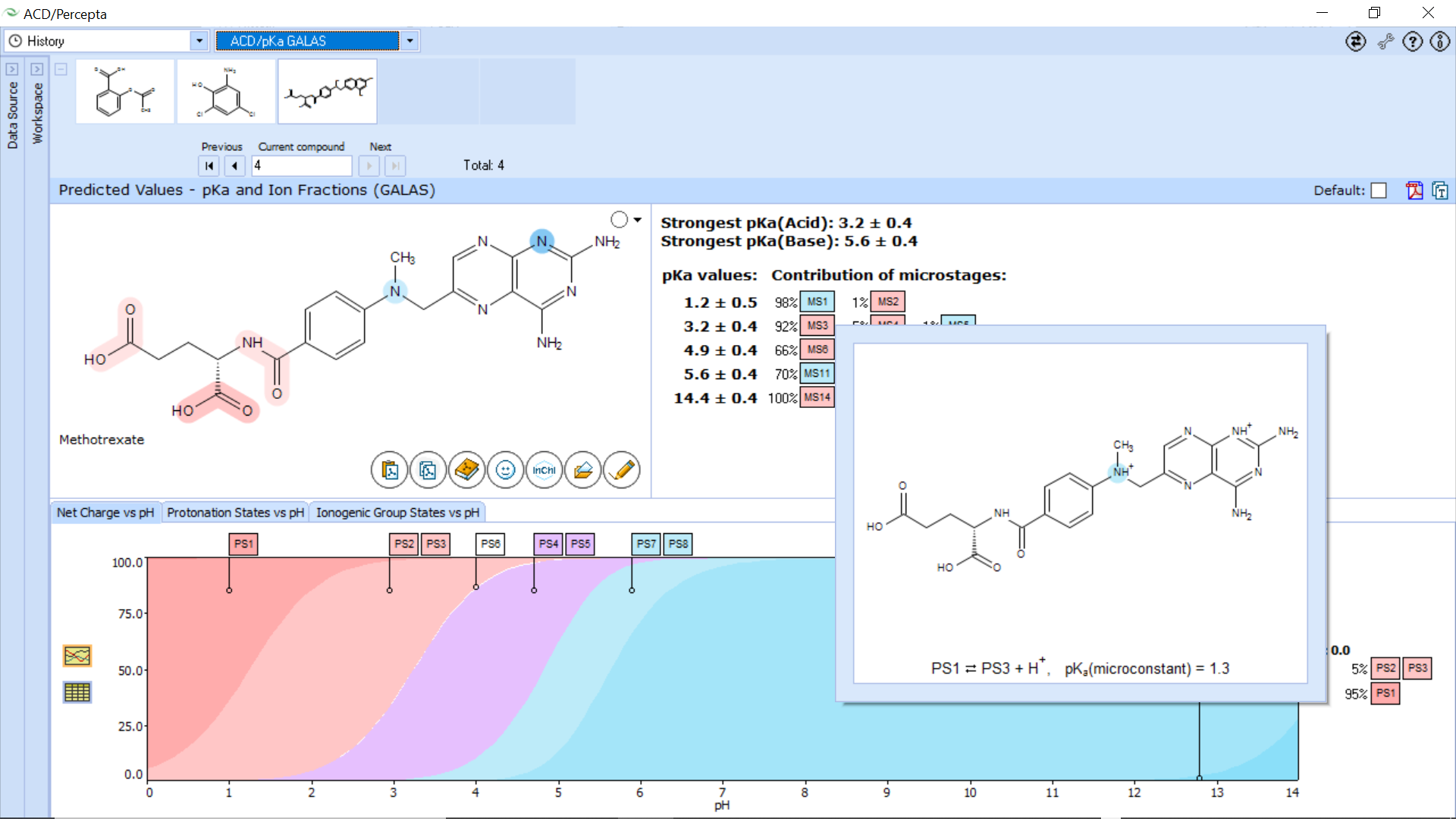
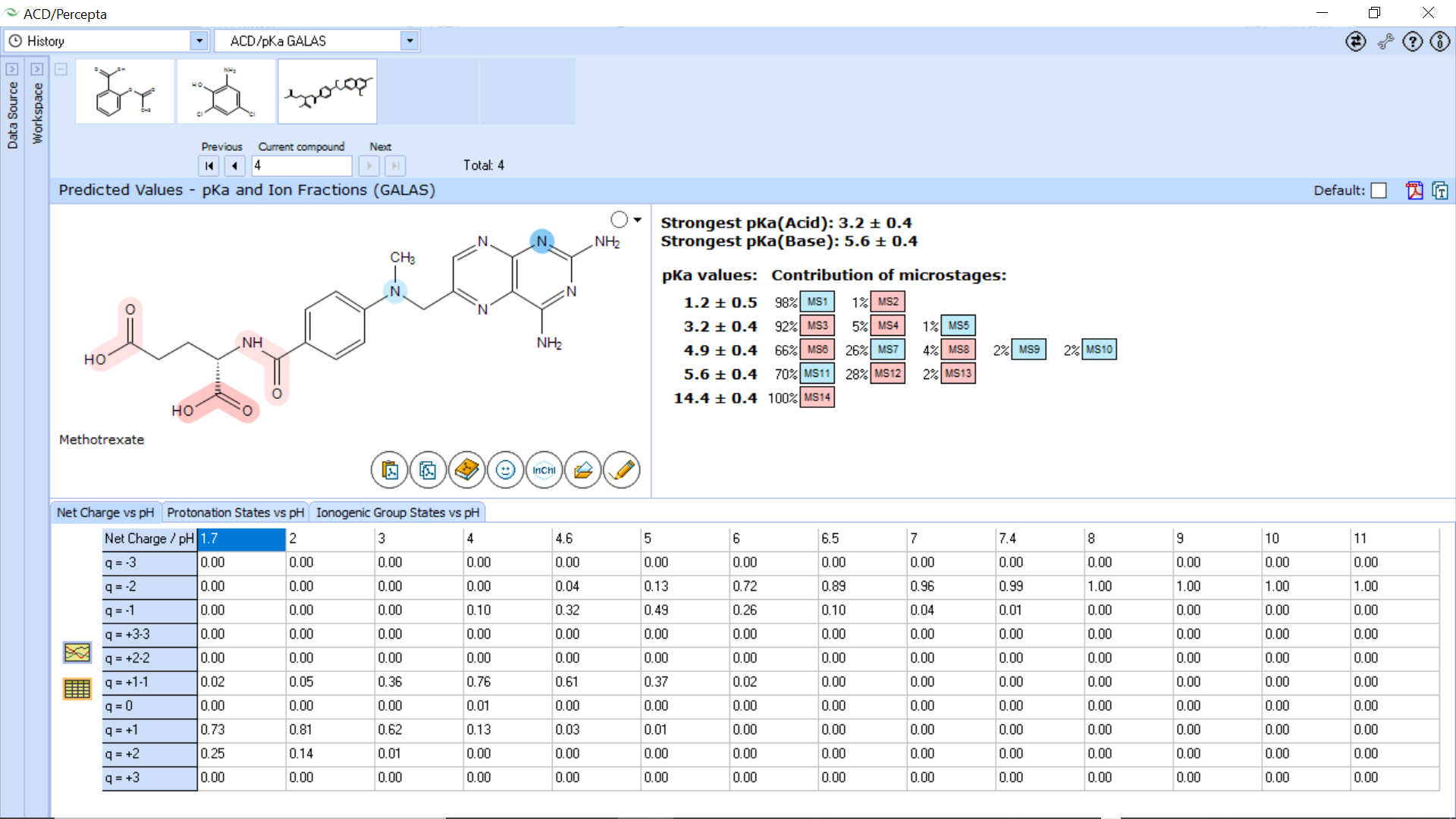
ACD/pKa is designed to predict pKa (acid dissociation constants) for organic molecules, providing valuable insights into their ionization behavior. It allows users to calculate pKa values under standard conditions, visualize all ionizable centers within a compound, and plot the distribution of ionized microspecies as a function of pH. The software also assesses the reliability of predicted pKa values, offering a reliability index, the five most similar structures from its database, and literature references for the original experimental data. A key benefit is the ability to train the algorithm with user's own experimental data, improving accuracy for specific chemical spaces. The software provides automatically generated plots of pKa versus pH, enabling users to identify trends and prioritize compounds. Color-mapping on the structure helps to easily identify ionizable groups and related structure fragments. The software combines results from two prediction algorithms and can be customized with in-house data to improve accuracy.
Features & Benefits
- Accurate, Reliable Results
- Predictions are based on a database of >26,000 compounds, continually expanded to improve accuracy. Evaluates accuracy with reliability index, similar structures, and literature references.
- Deeper Insights
- Assess the distribution of ionized states and microspecies from a variety of plots. Understand the behavior of your molecule with automatically generated plots of pKa versus pH. Identify trends and prioritize compounds easily—create scatter plots, browse, filter, sort, and rank libraries.
- Convenient Visualization
- Color-mapping on the structure helps you easily identify ionizable groups and the structure fragment related to each calculated pKa value. User-defined color-coding of results will help you quickly identify favorable and unfavorable entities in compound libraries.
- A 2-in-1 pKa Calculator
- Results from two prediction algorithms in one software application.
- Customizable with In-House Data
- Get the accuracy of an in-house model from a commercial product. Use your own experimental pKa data to expand the applicability domain to proprietary chemical space. Build a training set for each project for fine-tuned accuracy.
- General Features
- Calculate pKa from structure (draw in-app, or copy/paste from third-party drawing packages); SMILES string; InChI code; imported MOL, SK2, SKC, or CDX files; or search by name in the built-in dictionary. Two algorithms: ACD/pKa Classic (default calculator) and GALAS (Global, Adjusted Locally According to Similarity).
- Prediction Capabilities
- Prediction of the acid dissociation constant (pKa) under standard conditions (25°C, zero ionic strength) in aqueous solution for every ionizable group. Calculate pKa for organic molecules, metallo-organics, salts, hydrates, mixtures, proteins (MW ≤2000 Daltons), and polymeric units.
- Ionization Process Information
- Information provided about each ionization process (dissociation reaction) for all stages of ionization. Color-coding of ionizable groups to identify acidic, basic, and amphoteric ionization centers. Calculation of the strongest acid and base dissociation constants.
- Reliability and Protocol
- Estimation of the reliability of calculated pKa (in ±log units). Detailed calculation protocol for each predicted ionization (referred to as dissociation stage). Hover on a dissociation stage to see the related ionizable center highlighted on the structure. Click structure fragment to see it highlighted on the structure.
- Data Handling
- Calculate pKa properties for groups or libraries of compounds and use built-in tools to sort, filter, plot, and rank results. Set user-defined label colors. Filter results numerically. Sort results by ascending/descending values. See results for previously calculated values in the history. Report results to PDF or copy to your application of choice.
- Algorithm Training
- Train the algorithm with experimental data.