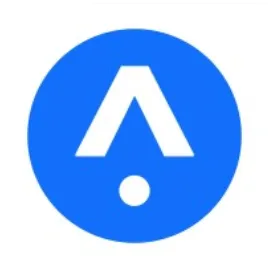
Synthetic DatasetsApplied Intuition
Accelerate ML model training with Applied Intuition's high-fidelity synthetic datasets.
Vendor
Applied Intuition
Company Website
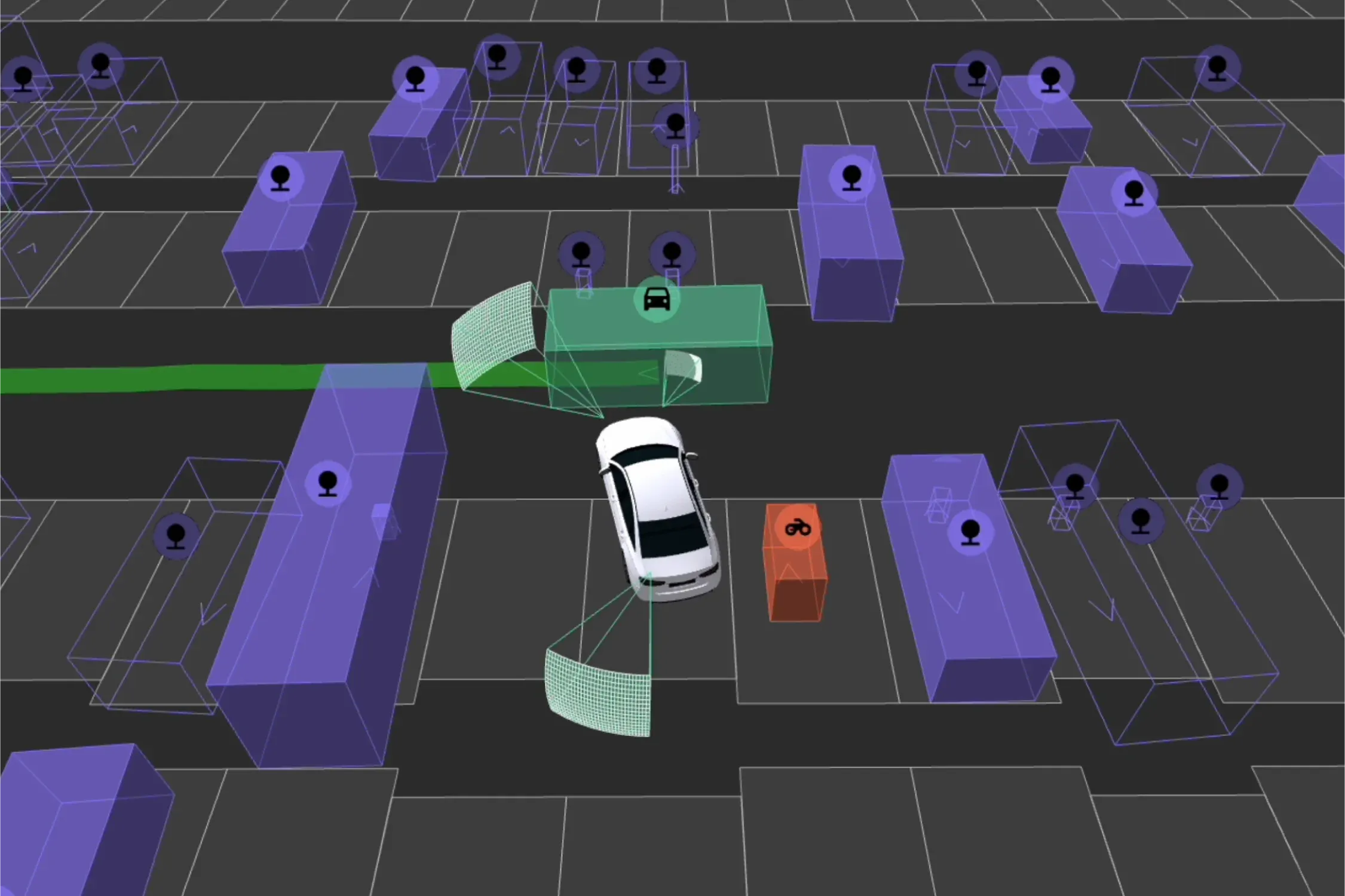
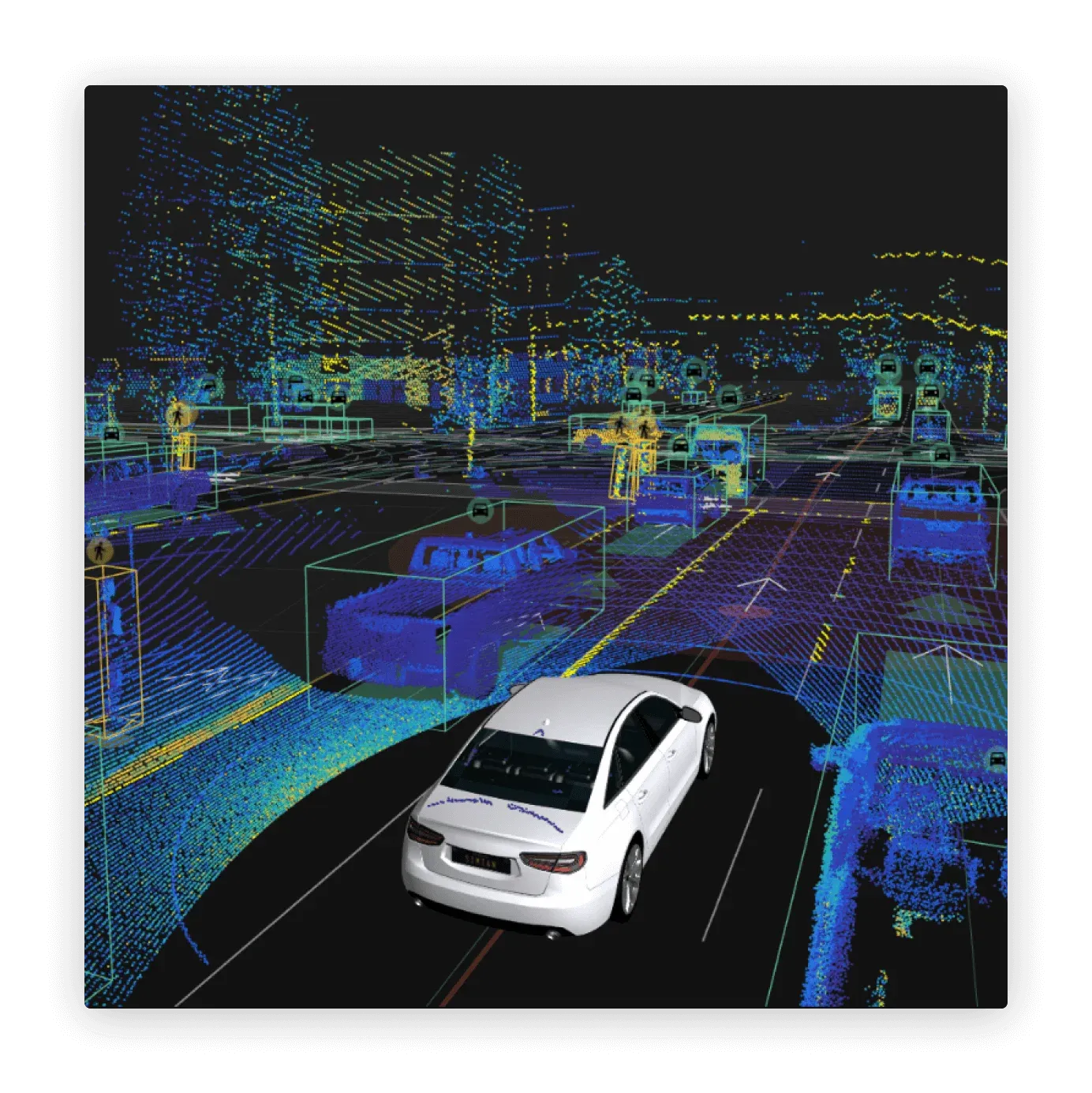
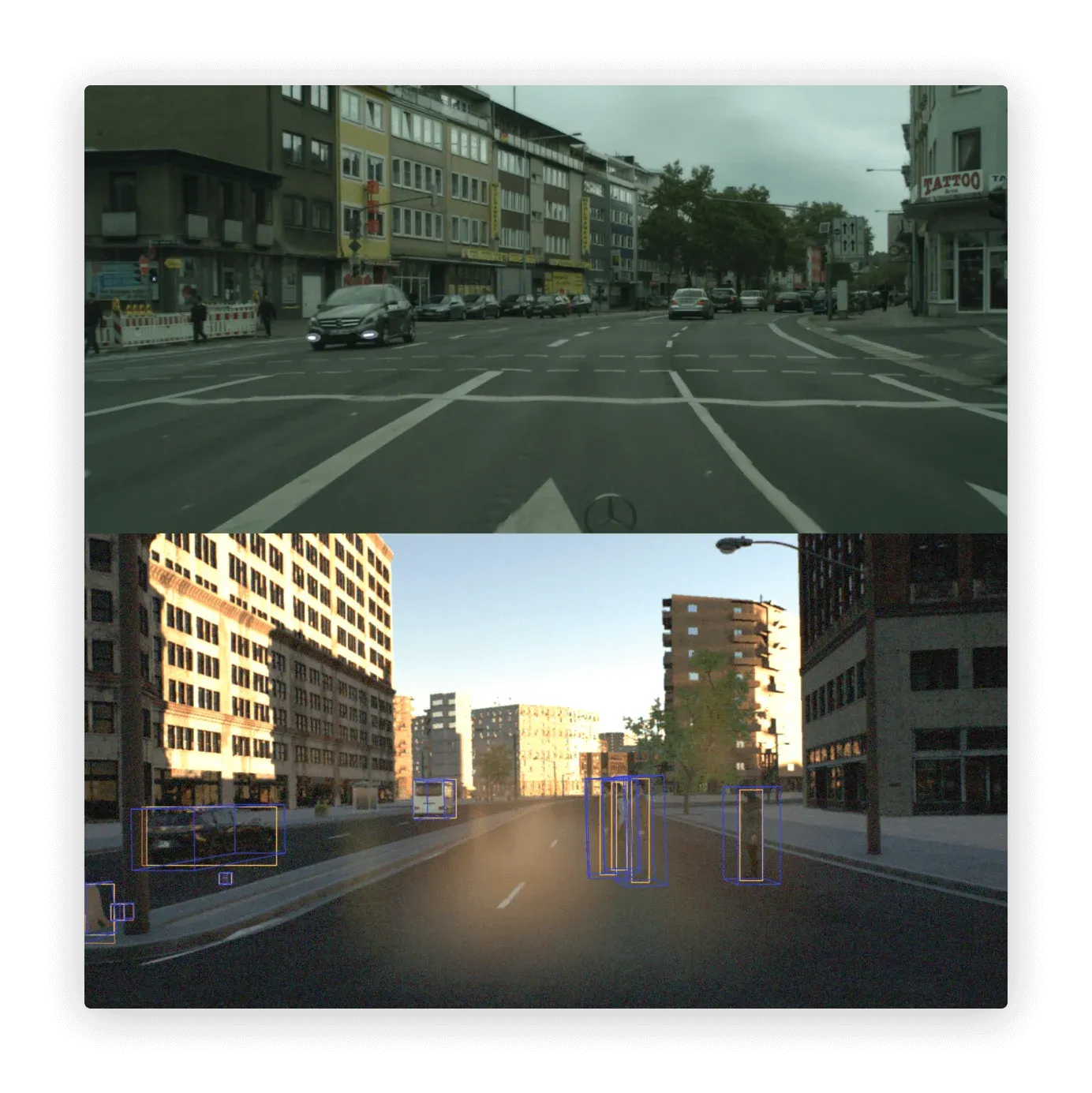
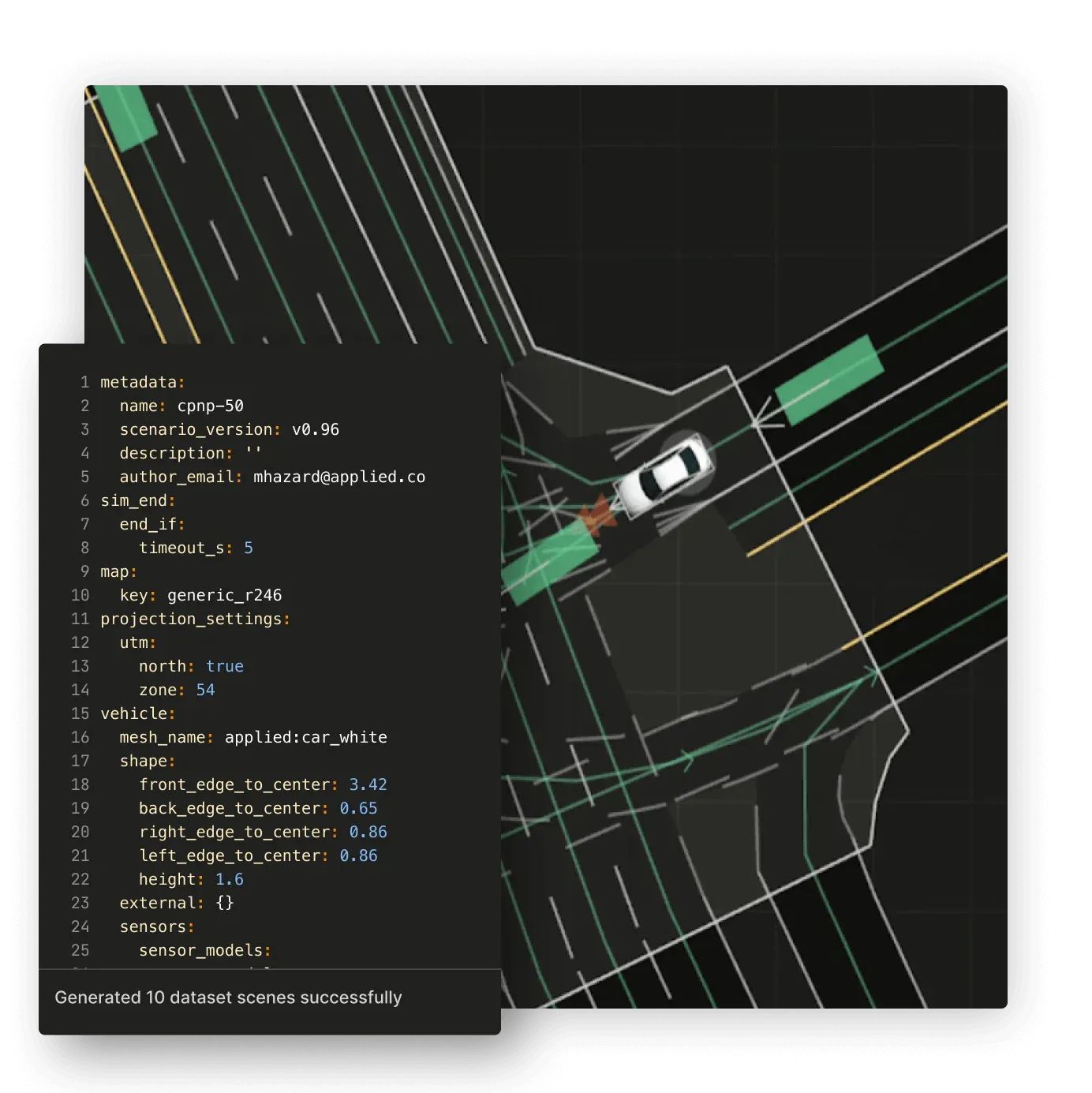
Product details
Overview
Applied Intuition's Synthetic Datasets offer a comprehensive solution for enhancing machine learning (ML) model training in advanced driver-assistance systems (ADAS) and automated driving (AD) applications. By generating high-realism synthetic sensor data, these datasets enable teams to develop and validate ML models more efficiently, reducing reliance on costly and time-consuming real-world data collection and labeling. Key components of this solution include rapid scene generation, sensor simulation, domain adaptation, and scalable infrastructure, all designed to streamline the development process and improve model performance.
Features and Capabilities
- Rapid Scene Generation: Define and generate synthetic datasets at scale using natural language scenario generation, a distribution-based domain randomization framework, or by extracting and augmenting scenes from real-world logs. This flexibility ensures datasets closely match specific task domains and target edge cases effectively.
- Sensor Simulation: Build upon Applied Intuition’s Sensor Sim to produce synthetic data that is physically accurate and representative of target sensors and task domains, ensuring diversity and realism necessary for effective ML training.
- Domain Adaptation: Modify synthetic data to align with real-world task domains through generative and classical algorithms, minimizing domain gaps and maximizing the value of synthetic datasets for ML systems.
- Scalable Infrastructure: Leverage Applied Intuition’s Cloud Engine to orchestrate thousands of parallel simulations, enabling the generation of production-scale datasets within hours.
- Parking Datasets: Provide labeled training data for parking-related perception tasks, such as parking spot detection and depth estimation, addressing class imbalances and expanding operational design domains.
- Traffic Sign Datasets: Offer diverse, physically accurate, and labeled training data for traffic sign recognition and classification, enhancing model robustness in traffic sign detection.
- Log-Based Testing: Enable rigorous validation of AV systems using real-world data within controlled simulation environments, enhancing reliability and safety before deployment.
- Action Recognition and Terrain Segmentation: Support challenging tasks like off-road terrain segmentation and action recognition from aerial assets, minimizing domain gaps and improving model performance.
Find more products by industry
Other ServicesManufacturingProfessional ServicesInformation & CommunicationView all