Cohere EmbedCohere
Embed is the leading multimodal embedding model. It acts as an intelligent retrieval engine for semantic search and retrieval-augmented generation (RAG) systems.
Vendor
Cohere
Company Website
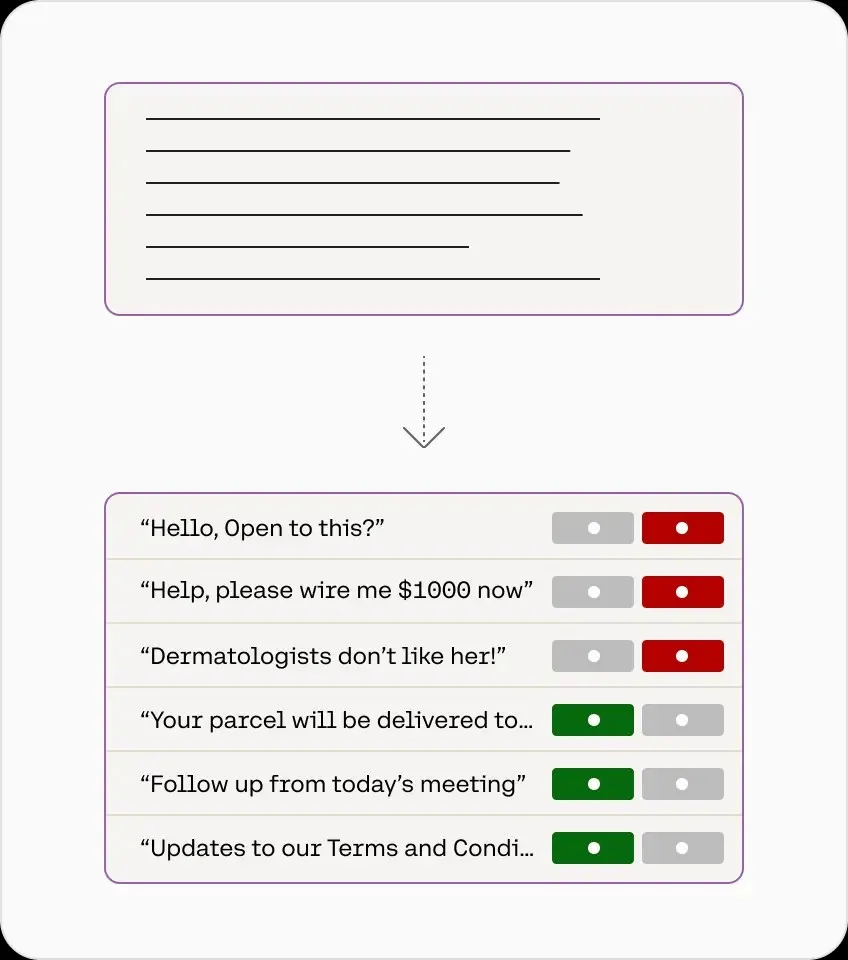
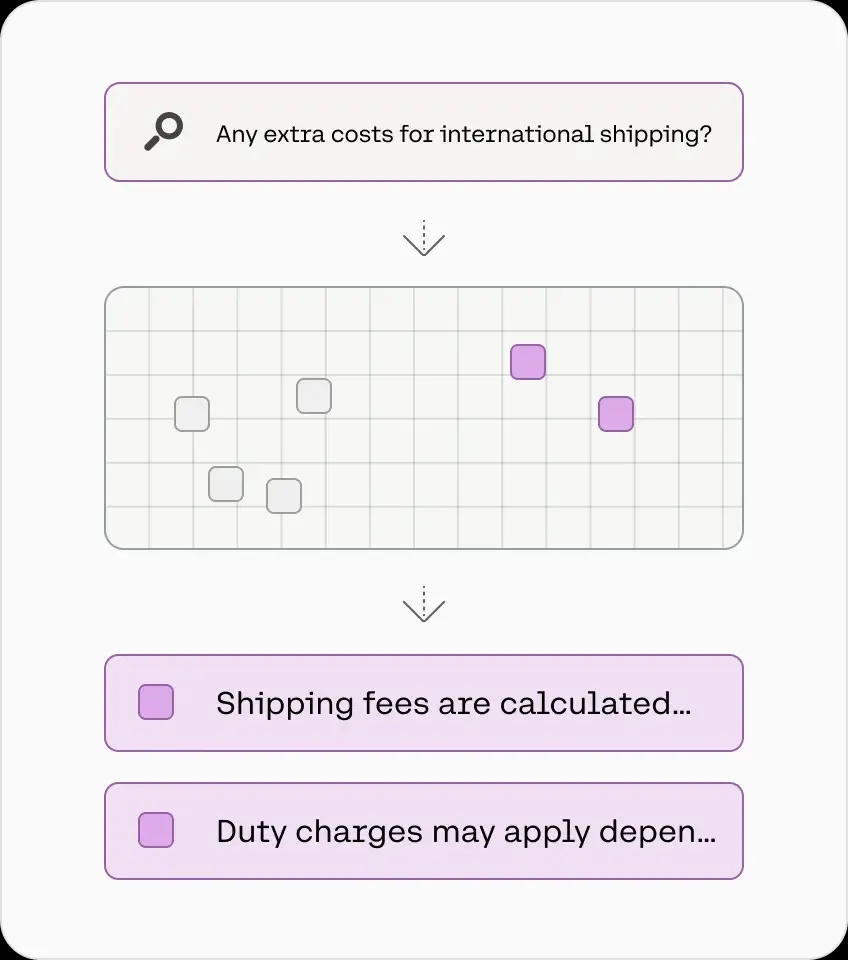
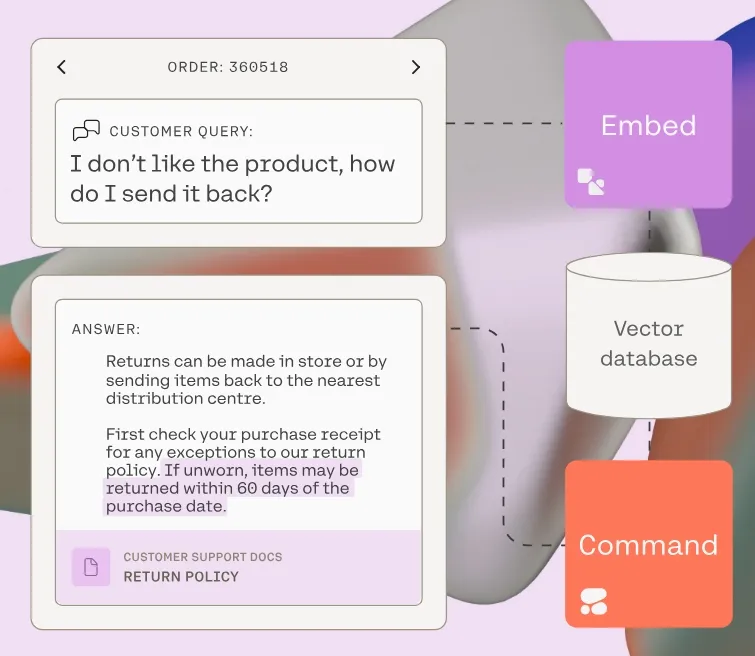
Product details
Overview
Cohere Embed is a leading multimodal embedding model designed to understand enterprise data. It functions as an intelligent retrieval engine, primarily used for semantic search and retrieval-augmented generation (RAG) systems. This model excels in converting data into embeddings, which are numerical representations capturing the semantic meaning of text and images. This allows for more effective and context-aware search capabilities compared to traditional keyword-based methods.
Features
- Multimodal Embeddings: Cohere Embed supports both text and image data, enabling a unified approach to understanding diverse types of information.
- Semantic Search: By leveraging embeddings, the model facilitates searching based on the meaning of the content, incorporating context and user intent more effectively than keyword matching.
- Retrieval-Augmented Generation (RAG): Embed is optimized for RAG systems, improving their performance by providing highly relevant search results.
- Clustering: The model can group similar texts and images based on their meaning, helping to uncover patterns in large datasets, such as common questions or similar issues.
- Text Classification: Cohere Embed enables the creation of systems that automatically classify text and image data into complex categories, streamlining tasks like ticket routing and content moderation.
- Robustness to Noisy Data: The model is designed to maintain high accuracy even when dealing with noisy, real-world datasets that contain errors or irrelevant information.
- Integration with Vector Databases: Embed is compatible with a wide range of vector databases, allowing seamless integration into existing workflows.
Benefits
- Improved Search Accuracy: Embed's semantic search capabilities provide more accurate and contextually relevant search results, enhancing information retrieval.
- Enhanced RAG Performance: The model's optimization for RAG systems leads to better retrieval of relevant information, saving time and computational resources.
- Data-Driven Insights: Clustering functionality helps uncover hidden patterns and relationships within large datasets, providing valuable insights for decision-making.
- Automated Content Classification: Text classification capabilities enable automated routing of tickets, moderation of content, and other tasks, improving efficiency.
- Efficient Data Handling: By understanding the nuances of data, even when noisy, Embed ensures reliable and accurate results.
- Streamlined Integration: Compatibility with various vector databases simplifies the process of incorporating Embed into existing systems.